OUR INTEREST
Investigating the complex network of multifaceted diseases with major global burden by integrating variants in multiple omic levels, biomarkers and environmental data collected in diverse populations, which may deliver a novel preventive approach, diagnoses, and treatment.
The Big Picture
Different genomic methodologies have been applied to examine the complex genetic architecture of metabolic diseases such as diabetes, cardiovascular diseases (CVD), and cancer. Increasing integration of cutting edge genomic science into population-based epidemiologic investigation has been not only accelerating and ameliorating the understanding of the genetic susceptibility of these complex traits, but also utilizing the identified genes for disease intervention and risk prediction. Here illustrates what we have been doing:
Research Area
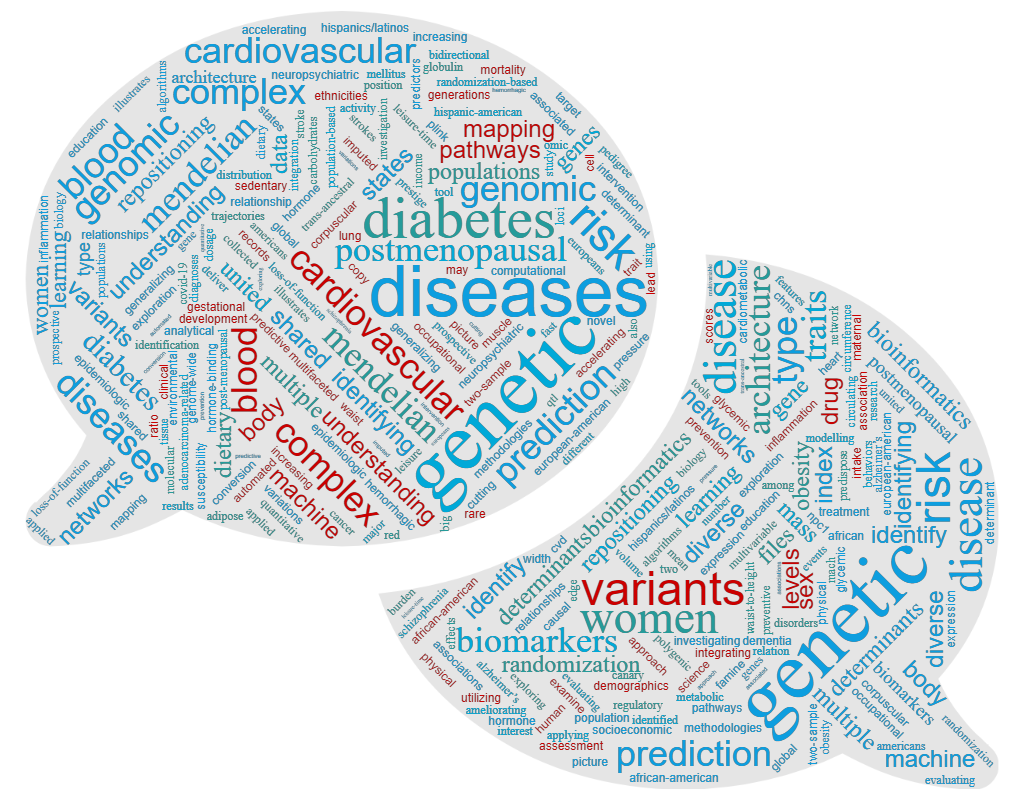